Polluted aquifer inverse problem solution using artificial neural networks
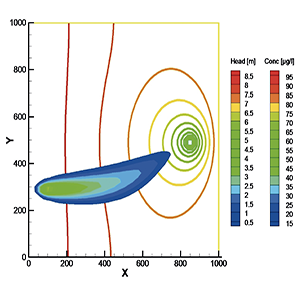
All claims expressed in this article are solely those of the authors and do not necessarily represent those of their affiliated organizations, or those of the publisher, the editors and the reviewers. Any product that may be evaluated in this article or claim that may be made by its manufacturer is not guaranteed or endorsed by the publisher.
The problem of identifying an unknown pollution source in polluted aquifers, based on known contaminant concentrations measurement in the studied areas, is part of the broader group of issues, called inverse problems. This paper investigates the feasibility of using Artificial Neural Networks (ANNs) for solving the inverse problem of locating in time and space the source of a contamination event in a homogeneous and isotropic two dimensional domain. ANNs are trained in order to implement an input-output relationship which associates the position. Once the output of the system is known, the input is reconstructed by inverting the trained ANNs. The approach is applied for studying a theoretical test case where the inverse problem is solved on the basis of measurements of contaminant concentrations in monitoring wells located in the studied area. Groundwater pollution sources are characterized by varying spatial location and duration of activity. To identify these unknown pollution sources, concentration measurements data of monitoring wells are used. If concentration observations are missing over a length of time after an unknown source has become active, it is more difficult to correctly identify the unknown pollution source. In this work, a missing data scenario has been taken into consideration. In particular, a case where only one measurement has been made after the pollutant source interrupted its activity has been considered.
Bashi-Azghadi S. N., Kerachian R., Bazargan-Lari M.R., Solouki K., 2010. Characterizing an unknown pollution source in groundwater resources systems using PSVM and PNN. Expert Systems with Applications. DOI: https://doi.org/10.1016/j.eswa.2010.04.019
Carcangiu S., Di Barba P., Fanni A., Mognaschi M.E., Montisci A., 2007. Comparison of multi objective optimisation approaches for inverse magnetostatic problems. COMPEL: Int. J. for Computation and Maths in Electrical and Electronic Eng., Vol. 26, N. 2, pp. 293-305. DOI: https://doi.org/10.1108/03321640710727656
Cybenko G., 1989. Approximation by Superposition of a Sigmoid Function. Mathematics for Control, Signals and Systems. Vol.2, pp. 303-314. DOI: https://doi.org/10.1007/BF02551274
Fanni A., Uras G., Usai M., Zedda M.K., 2002. Neural Network for monitoring groundwater. Fifth International Conference on Hidroinformatics, Cardiff, UK, pp. 687-692.
Fanni A., Montisci A., 2003. A Neural Inverse Problem Approach for Optimal Design. IEEE Transaction on Magnetics, Vol. 39, N. 3, pp. 1305 1308. DOI: https://doi.org/10.1109/TMAG.2003.810541
Foddis M.L., Montisci A., Uras G., Ackerer P. . 2012. Ann based approach to solve groundwater pollution inverse problem. 9th International Conference of Modeling, Optimization and Simulation - MOSIM’12, Bordeaux, France. 06-08 June 2012.
Gümrah F., Öz B., Güler B. And Evin S., 1990. The application of artificial neural networks for the prediction of water quality of polluted aquifer. Water, Air, and Soil Pollution, Vol. 119, pp. 275–294. DOI: https://doi.org/10.1023/A:1005165315197
Mahar P. S. and Datta B., 2000. Identification of Pollution Sources in Transient Groundwater Systems. Water Resources Management, Vol. 14, Issue 3, pp 209-227. DOI: https://doi.org/10.1023/A:1026527901213
Rajanayaka C., Samarasinghe S. & Kulasiri D., 2002. Solving the Inverse Problem in Stochastic Groundwater Modelling with Artificial Neural Networks. Available on line at http://www.iemss.org/iemss2002/proceedings/vol2.html.
Rizzo, D.M. and D.E. Dougherty, 1994. Characterization of aquifer properties using artificial neural networks: Neural Kriging. Water Resources Research. Vol. 30, N.2, pp. 483-497. DOI: https://doi.org/10.1029/93WR02477
Sciuntu C., PhD thesis, 2004 . Reti neurali artificiali: una applicazione nello studio di acquiferi contaminati. University of Cagliari.
Singh R. M. and Datta B., 2006. Artificial neural network modeling for identification of unknown pollution sources in groundwater with partially missing concentration observation data. Water Resources Management. Vol. 21, pp. 557-572. DOI: https://doi.org/10.1007/s11269-006-9029-z
Zhiqiang L., Rizzo D. and Hayden N., 2006. Utilizing Artificial Neural Networks to Backtrack Source Location. Available on line at http://www.iemss.org/iemss2006/papers/s2/175_Li_2.pdf.
Zio E., 1997. Approaching the inverse problem of parameter estimation in groundwater models by means of artificial neural networks. Progress in Nuclear Energy, Elsevier Science. Vol 31, N.3, pp. 303-315. DOI: https://doi.org/10.1016/S0149-1970(96)00013-3
Copyright (c) 2022 the Author(s)

This work is licensed under a Creative Commons Attribution-NonCommercial 4.0 International License.
PAGEPress has chosen to apply the Creative Commons Attribution NonCommercial 4.0 International License (CC BY-NC 4.0) to all manuscripts to be published.